Variance Of Y Hat In Regression
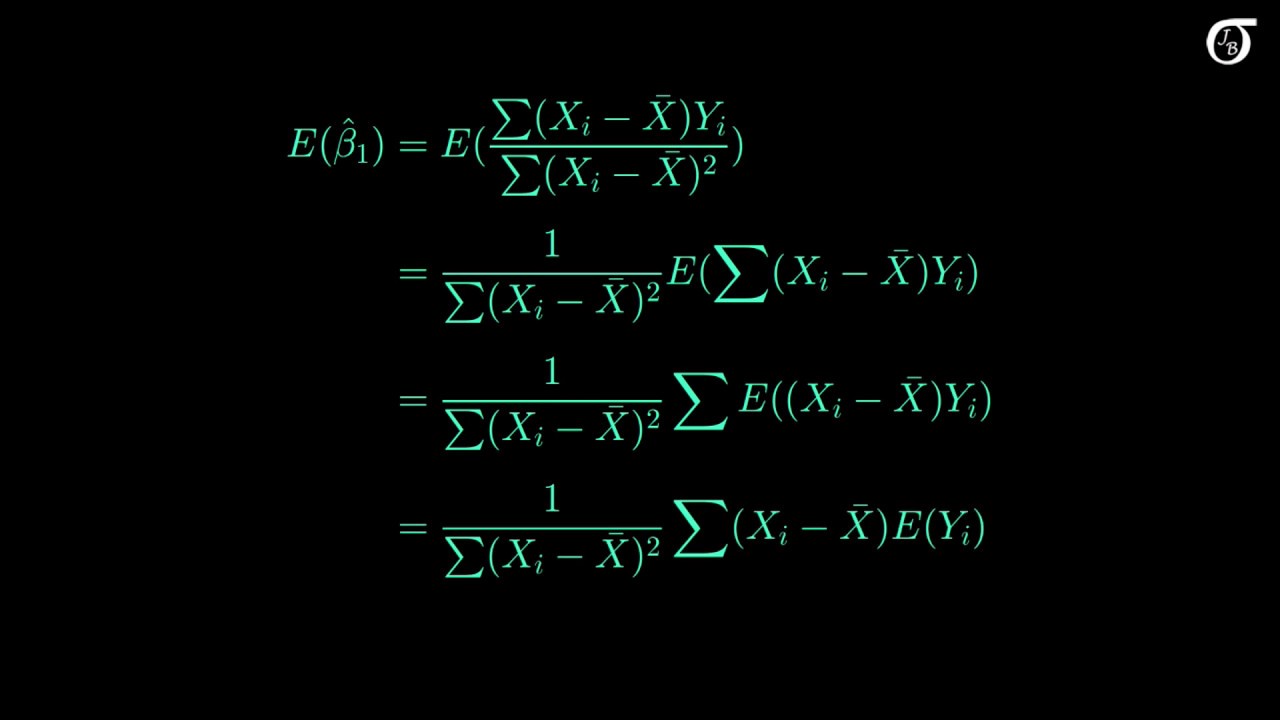
0Because there is conditional variability inY the scores onYcannot generally be perfectlypredicted from those onX and.
Variance of y hat in regression. The estimated alpha 497 and beta 106. In a regression model where E2i 0 and variance V2i 2 1 and 2i and 2j are uncorrelated for all i and j the least squares estimators b0 and b1 and unbiased and have minimum variance among all unbiased linear estimators. In doing so the unbiasedne.
-1 - 0 - 1 R2 Coefficient of Determination RR 100. X i is one single non-random variable so on itself it has a variance of 0 so the formula you wrote simplifies to just σ 2. We derive the mean and variance of beta hat the Estimated Regression Parameter vector in a Full Rank Gauss-Markov Linear Model.
That SSTO SSR SSE. Regression models predict a value of the Y variable given known values of the X variables. Remember b 1 P X i X Y i Y P X i X 2 b 0 Y b 1X.
Regression constant in these simpler models will help us to understand both the constant and the other regression coefficients in later more complex models. At the hat matrix can reveal sensitive points in the design points at which the value of y has a large impact on the fit Huber 1975. Please like comment subscribePLEASE SUBSCRIBE.
Y-hat a b1X1 b2X2 b3X3 b4X4 Y-hat is the Dependent Variable X1 X2 X3 X4 are the Predictor Independent Variables College GPA-hat a b1HSGPA b2SAT b3ACT b4HoursWork R Multiple Correlation Range. They tell us that most of the variation in the response y SSTO 18276 is just due to random variation SSE 17085 not due to the regression of y on x SSR 1191. The hat matrix in regression is just another name for the projection matrix.
Essentially the model says that conditional mean ofYis linear inX with an intercept ofand a slope of 1 while the conditional variance is constant. The hat matrix is de ned as H X0X 0X 1X because when applied to Y it gets a hat. Prediction outside this range of the data is known as extrapolation.