Y Hat In Logistic Regression
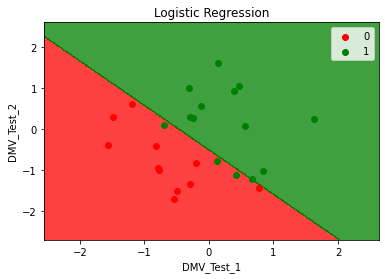
Logistic Regression not only gives a measure of how relevant a predictor coefficient size is but also its direction of association positive or negative.
Y hat in logistic regression. It is needless to say that logistic regression is one of the most straightforward yet very powerful classification machine learning algorithms under the umbrella of a supervised learning algorithm. For example the output can be SuccessFailure 01 TrueFalse or YesNo. It is used for predicting the categorical dependent variable using a given set of independent variables.
Like all regression analyses the logistic regression is a predictive analysis. Logistic regression assumes that the observations in the dataset are independent of each other. This is like a question that we can answer with either yes or no We only have two classes.
Binary logistic regression is the statistical technique used to predict the relationship between the dependent variable Y and the independent variable X where the dependent variable is binary in nature. That is the observations should not come from repeated measurements of the same individual or be related to each other in any way. What is is when to use it.
To predict discrete valued outcome. Logistic regression is a machine learning method used in the classification problem when you need to distinguish one class from another. The result is the impact of each variable on the odds ratio of the observed event of interest.
Logistic regression can be used to classify an observation into one of two classes like positive sentiment and negative sentiment or into one of many classes. It is a statistical approach that is used to predict the outcome of a dependent variable based on observations given in the training set. Logistic regression is one of the most popular Machine Learning algorithms which comes under the Supervised Learning technique.
We are precisely dealing with our regression line as we can see the term y into the equations. We see that Logistic regression is easier to implement interpret and very efficient to train. Logistic regression is the appropriate regression analysis to conduct when the dependent variable is dichotomous binary.